Understanding Machine Learning Data Labeling for Business Efficiency
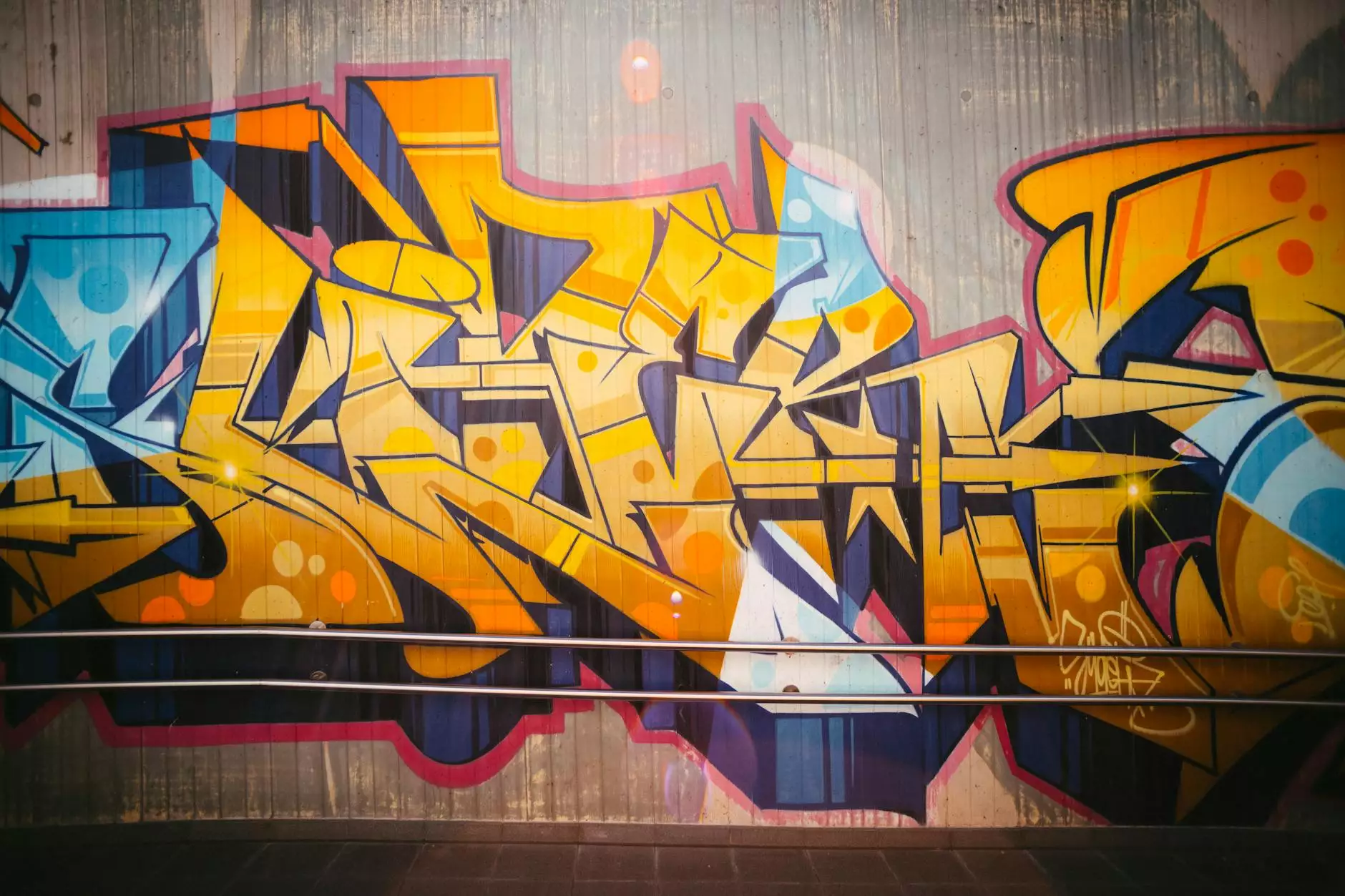
In today's fast-paced digital landscape, businesses are increasingly relying on machine learning data labeling to enhance their operational efficiency and decision-making processes. This practice is especially relevant in industries such as Home Services and Keys & Locksmiths, where accurate data can lead to improved customer service, streamlined operations, and ultimately, greater profitability.
The Role of Machine Learning in Business
Machine Learning (ML) is a subset of artificial intelligence that focuses on the development of algorithms that can learn from and make predictions based on data. The power of machine learning lies in its ability to process vast amounts of information quickly and extract valuable insights, which can significantly impact business strategies. However, for ML algorithms to be effective, the data they utilize must be accurately labeled.
What is Data Labeling?
Data labeling is the process of annotating data so that machine learning algorithms can understand and learn from it. This involves tagging images, videos, text, or audio with relevant labels that provide context. For instance, in a home services context, labeling might include tagging images of home repairs or locksmith jobs to provide contextual learning for a service recommendation engine.
Importance of Data Labeling in Machine Learning
Data labeling serves as the foundation upon which machine learning models are built. Here’s why it is crucial:
- Accuracy: Properly labeled data enhances the accuracy of ML algorithms. The more precise the labels, the better the model's ability to make correct predictions.
- Training Sets: Adequately labeled datasets are essential for training machine learning models. Without sufficient training data, models may not perform effectively in real-world scenarios.
- Automation: Data labeling is a step toward automating business processes. Accurate data allows systems to operate with minimal human intervention, reducing costs and increasing efficiency.
Types of Data Labeling
Machine learning data labeling can take various forms, depending on the type of data being processed. Here are some common types:
- Image Annotation: Used extensively in visual recognition projects. Images are tagged with descriptive labels, essential for training computer vision models.
- Text Annotation: Involves tagging text data for sentiment analysis, category classification, and entity recognition. In the locksmith industry, this could mean categorizing customer reviews or inquiries.
- Audio Annotation: Important for speech recognition technologies. Audio files may be labeled to identify keywords or customer requests within calls.
- Video Labeling: Involves labeling keyframes in video to teach models about motion detection, crucial for security services in home automation.
Benefits of Machine Learning Data Labeling for Home Services and Locksmiths
In the realms of Home Services and Keys & Locksmiths, machine learning data labeling can offer numerous benefits that significantly enhance business operations. Here are some key advantages:
1. Enhanced Customer Experience
By leveraging machine learning data labeling, businesses can refine their customer interaction processes. For example, a locksmith company can analyze service calls using labeled audio data to identify commonly asked questions and develop comprehensive FAQs or automated response systems, leading to a more efficient customer experience.
2. Better Service Recommendations
Data labeling enables machine learning algorithms to understand customer preferences and behavior. In a home service context, accurately labeled data can help companies suggest the most relevant services to customers based on their previous interactions and preferences, thereby increasing conversion rates.
3. Improved Operational Efficiency
By implementing machine learning solutions powered by well-labeled data, companies can optimize their operations. For instance, a locksmith business can analyze job data to predict busy periods and allocate resources more effectively, reducing wait times for customers.
4. Fraud Detection
Machine learning can also be applied to detect fraudulent activities in transactions. By labeling historical transaction data as legitimate or fraudulent, algorithms learn to distinguish between normal and suspicious activities, helping businesses mitigate risks.
Challenges in Data Labeling
While the benefits are significant, businesses must also navigate challenges associated with machine learning data labeling:
- Quality Control: Ensuring the quality of labeled data can be challenging. Mislabeling can lead to incorrect predictions and poor decision-making.
- Scalability: As businesses grow, the volume of data that needs to be labeled often increases dramatically, making it essential to establish efficient labeling processes.
- Resource Intensive: Data labeling can be time-consuming and labor-intensive, requiring skilled personnel or advanced tools to automate the process.
Best Practices for Effective Data Labeling
To overcome these challenges, businesses should adopt best practices for data labeling:
- Clearly Define Labels: Ensure that all labels used in data annotation are well-defined, reducing ambiguity and enhancing accuracy.
- Utilize Automation Tools: Implement data labeling tools that leverage automation to speed up the labeling process without compromising quality.
- Regular Audits: Conduct periodic reviews of labeled data to identify inconsistencies and correct them, thus maintaining a high standard of data quality.
- Continuous Learning: Update labeling guidelines based on model performance feedback to keep improving the accuracy of the labeling process.
Conclusion
The implementation of machine learning data labeling is revolutionizing how businesses, especially in the Home Services and Keys & Locksmiths sectors, operate. By investing in quality data labeling practices, organizations can enhance customer experiences, improve service delivery, and achieve significant operational efficiencies. As the digital landscape continues to evolve, mastering data labeling will be integral to staying ahead of the competition and ensuring business growth.
In conclusion, businesses that harness the power of machine learning, supported by well-executed data labeling techniques, will undoubtedly gain a competitive edge in the market. The future is data-driven, and those who invest wisely today will reap the rewards tomorrow.